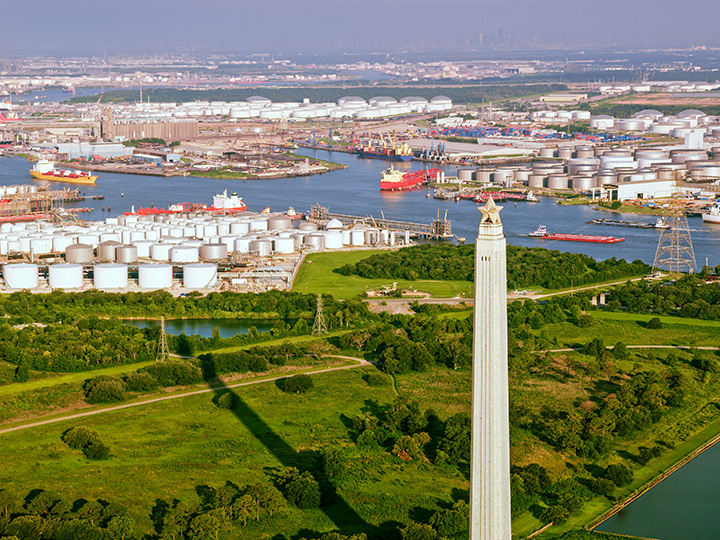
Project Summary
Houston faces significant air pollution challenges, particularly with surface ozone, which can negatively impact health. A University of Houston research team has leveraged machine learning (ML) and innovative analysis techniques to more accurately identify the sources of ozone pollution in the city.
Methods
The team combined the Positive Matrix Factorization (PMF) model with the SHAP algorithm, an ML tool that enhances interpretability, to analyze ozone emissions in both industrial and urban areas of Houston. Their study revealed that the oil and gas industry significantly influences ozone pollution in industrial areas, while shortwave radiation and humidity play key roles in ozone concentration levels citywide.
Using multi-year VOC data from Texas Commission on Environmental Quality monitoring stations, the researchers compared emissions from the Houston Ship Channel, a major industrial site, and Milby Park, a typical urban area. Their two-step modeling approach identified clear chemical differences between these areas, helping to understand the distinct pollution sources affecting Houston.
Goals
This research offers valuable insights for local policymakers to develop targeted emission reduction strategies, ultimately leading to cleaner air and improved public health. The team also plans to expand this approach to other cities, with the aim of tailoring pollution control strategies to the unique characteristics of each area.